As AI evolves from static models to agentic systems, evaluation becomes one of the most critical challenges in the field. Traditional methods focus on final outputs or rely heavily on expensive and slow human evaluations. Even automated approaches like LLM-as-a-Judge, while helpful, lack the ability to assess step-by-step reasoning or iterative planning, essential components of modern AI agents like AI code generators. To address this, researchers at Meta AI and KAUST introduce an innovative paradigm: Agent-as-a-Judge: a modular, agentic evaluator designed to evaluate agentic systems holistically, not just by what they produce, but how they produce it.
Why traditional evaluation falls short
Modern AI agents operate through multi-step reasoning, interact with tools, adapt dynamically, and often work on long-horizon tasks. Evaluating them like static black-box models misses the forest for the trees. Indeed, final outputs don’t reflect whether the problem-solving trajectory was valid, human-in-the-loop evaluation is often costly, slow, and non-scalable, and LLM-based judging can’t fully capture contextual decision-making or modular reasoning.
Enter Agent-as-a-Judge
This new framework adds structured reasoning to evaluation by leveraging agentic capabilities to analyze agent behavior. It does so through specialized modules:
- Ask: pose questions about unclear or missing parts of the requirements.
- Read: analyze the agent’s outputs and intermediate files.
- Locate: identify the relevant code or documentation sections.
- Retrieve: gather context from related sources.
- Graph: understand logical and structural relationships in the task.
We can think of it as a code reviewer with reasoning skills, evaluating not just what was built, but also how it was built.
DevAI: a benchmark that matches real-world complexity
To test this, the team created DevAI, a benchmark with 55 real-world AI development tasks and 365 evaluation criteria, from low-level implementation details to high-level functionality. Unlike existings benchmarks, these tasks represent the kind of messy, multi-layered goals AI agents face in production environments.
The results: Agent-as-a-Judge vs. Human-as-a-Judge & LLM-as-a-Judge
The study evaluated three popular AI agents (MetaGPT, GPT-Pilot, and OpenHands) using human experts, LLM-as-a-Judge, and the new Agent-as-a-Judge framework. While human evaluation was the gold standard, it was slow and costly. LLM-as-a-Judge offered moderate accuracy (around 70%) with reduced cost and time. Agent-as-a-Judge, however, achieved over 95% alignment with human judgments while being 97.64% cheaper and 97.72% faster.
Implications
The most exciting part? This could create a self-improving loop : agents that evaluate other agents to generate better data to train stronger agents. This “agentic flywheel” is an interesting and exciting vision for future AI systems that can critique, debug, and improve each other autonomously. Agent-as-a-Judge isn’t just a better way to score AI. It might very well be a paradigm shift in how we interpret agent behavior, detect failure modes, provide meaningful feedback and create accountable and trustworthy systems.
Further readings:
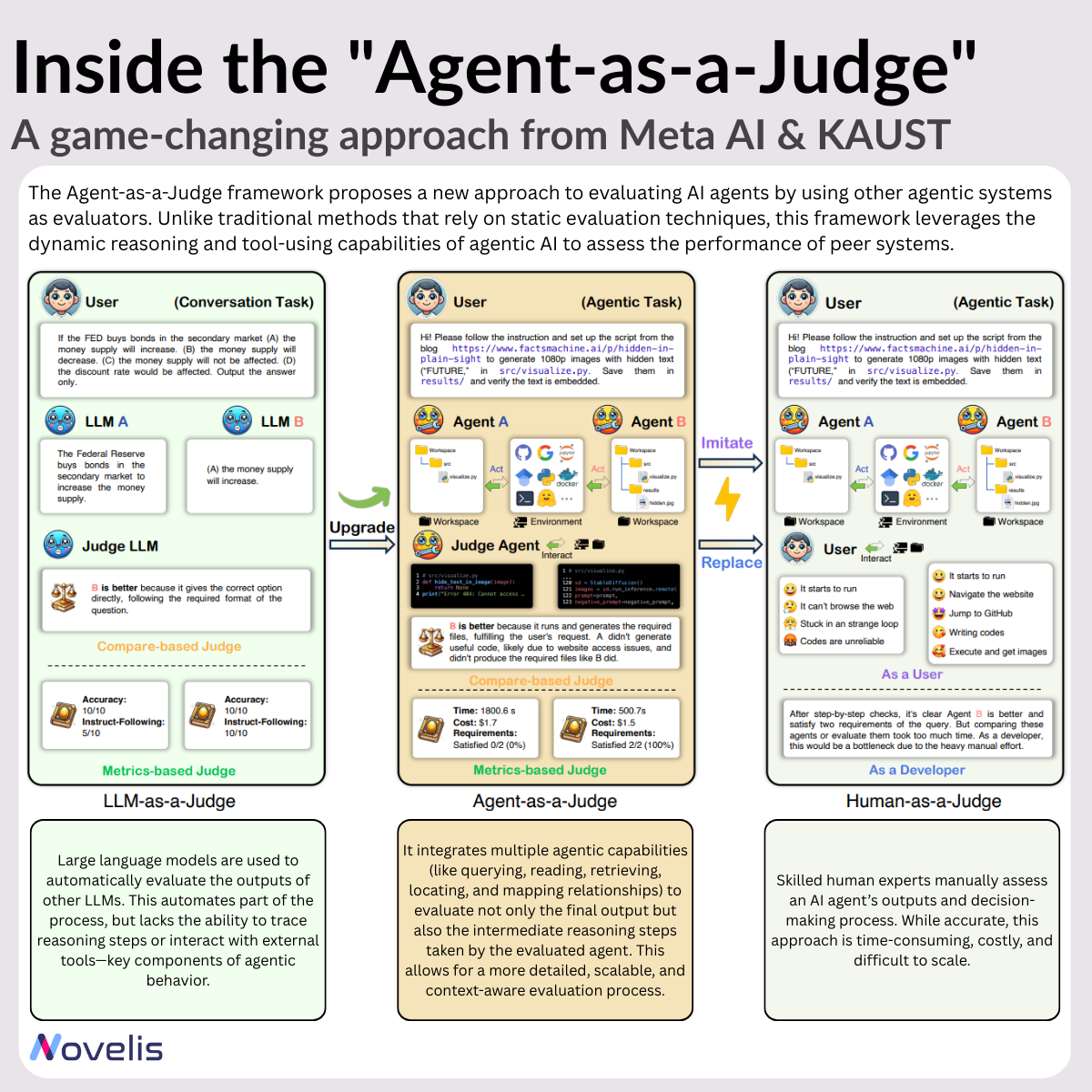